LAKE – FREVO
ID: R18 | Licence: Apache 2.0 | Owner: LAKE | Contacts: schranz@lakeside-labs.com |
Short Description | FREVO creates local rules for MIRTO edge/fog agents or pods using evolutionary algorithms. Therefore, FREVO needs to be connected to a simulation framework (COSMOS, DynAA). |
Key features | – Modular structure, easily extendable – Contains several neural network models and evolutionary algorithms |
Require | FREVO requires the Java environment (version min. 1.6) to be installed. |
Provide | FREVO comes with the following NN representation options: • Fully-meshed net: A time-discrete, recurrent ANN where each neuron is connected to every other neuron and itself. During evolution, the biases of each neuron, plus the connection weights are taken into account. • Three-layered net: A similar ANN to the previous one, but with a feed-forward structure instead of the fully-meshed one. • NEAT net: An ANN whose connectivity structure is also taken into account for selection during evolution. • Econets: Neural networks that can learn from their previous outputs. • MealyFSM: A Mealy Machine (a special case of a Finite State Machine) whose structure and transition probabilities are evolved. |
Input | The input is Interface specific, for instance for COSMOS we have a main config file, that allows to run the FREVO training: – num_pods: 2500 # must be bigger than or equal to sim_steps – pods_per_step: 0 # set to 0 for the default value (num_pods/sim_steps) – sim_steps: 10 – num_edge_devices: 35 # ignored if import_sample is True – num_fog_nodes: 10 # ignored if import_sample is True – num_cloud_servers: 5 # ignored if import_sample is True – behaviour_type: ‘mlp’ # ‘random_walk’, ‘aco’ or ‘mlp’ (for neural network based decision-making) – import_graph: True – graph_sample: EFC1 – nn_sample: ‘mlp_config15:31:57.462020.yaml’ # needs to be specified for ‘mlp’ behaviour_type mode ‘normal’ – plot_all: True # takes effect if mode is not ‘train’ – gen_samples_num: 50 # takes effect if mode == ‘gen’ – mode: ‘train’ # ‘normal’ for normal mode, ‘gen’ for generating samples, ‘train’ for training – path_to_dataset: ‘generated/dataset’ – frevo_port: 9990 – candidate_evals: 10 # required number of inference requests to evaluate a candidate – training_results_path: “/home/madnad/Documents/Myrtus/Frevo/Frevo/Results/EFC_test” # to save the best trained model – evaluate_best_candidate: True # enables running simulation with the best trained model after training session – randomized_pod_interval: True # if True, the pods will have a random arrival interval – mu: 0.5 # parameter µ ∈ (0, 1] defining the frequency of arriving pods – training_results_path: “/home/user/Documents/Myrtus/Frevo/Frevo/Results/EFC_test” – evaluate_best_candidate: True – randomized_pod_interval: False – mu: 0.5 |
Output | The interface has a continuous loop to follow, as shown in Figure 1. Information about algorithmic evaluation can be found in COSMOS description. |
User | System designers Researchers |
Benefits for the user | Possibility to design algorithms based on evolution for the MYRTUS 360° vision. |
Position in the MYRTUS DPE | Step 1 – Continuum modeling, simulation and analysis |
TRL@M0 | 3 |
TRL@M36 | 4-5 |
General description
FREVO is a framework for evolutionary design and offers multiple options for candidate representations (incl. fuzzy rules, FSM or ANN) as well as optimization method parameter selections. In several papers and implementations we were able to show the stability and the diverse possibilities for the application of FREVO to generate rules (even for swarms) using genetic manipulations.
Role in the MYRTUS DPE
In MYRTUS, we create an interface to an existing simulation framework (COSMOS, DynAA probably in future). FREVO needs an external simulation tool to allow to produce and evaluate the many generations for working on evolutionary algorithms.
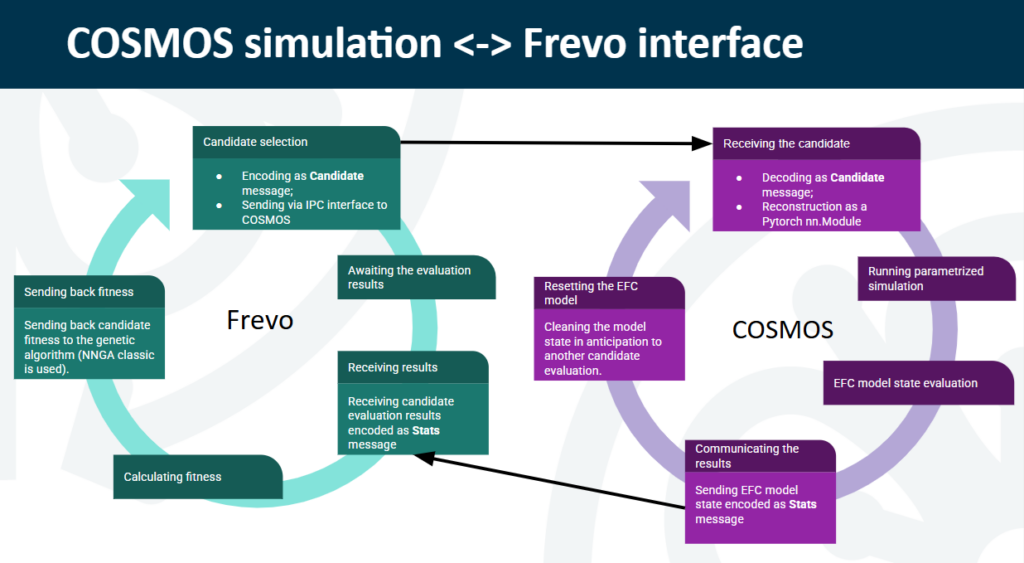
MYRTUS Extension/Contribution
In MYRTUS we use FREVO to design rules for the WL manager based on evolutionary algorithms. As FREVO needs a simulation tool for mutating, selecting, generating candidate solutions (computed by evolutionary algorithms), we need a new interface ( to DynAA or to the simulation framework designed to evaluate the swarm-based orchestration) to simulate the MYRTUS 360° vision to generate precisely such rules in combination with genetic manipulation.
Plans and Expectation
Assessment Plan@M18:
We have already derived all the requirements for the FREVO interface and finished the interface implementation to COSMOS. For the assessment, we have established a first simulation loop with FREVO to create swarm behaviour with evolution.
Expected Results@M18:
Implementation of one interface to FREVO and get first results in the design of swarm behaviour with this tooling loop (FREVO-COSMOS).